library(tidyverse)
library(httr)
library(jsonlite)
library(rlist)
library(knitr)
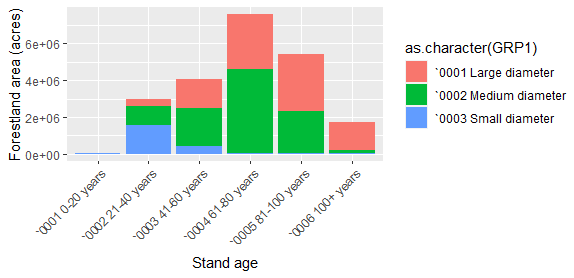
If you work with forestry data across the United States, you’ve probably had to turn to Forest Inventory and Analysis (FIA) data at one time or another to look at the status and trends in our forest resources. The FIA database has information contained from over 130,000 forest inventory plots across the country.
One of the tools that analysts often use to access FIA data is the the EVALIDator web interface. EVALIDator is a web-based Application Programming Interface (API) that generates population estimates for core forestry metrics. It provides the ability to query FIA information at the state level or a circular retrieval of information around a fixed geographic point.
There was an excellent webinar offered in August hosted by the FIA National User Group that highlighted some new features available through EVALIDator. For a long while, analysts have used it’s web interface to point and click their way to estimating forest resources after selecting the forestry metrics and geographic scale they’re interested in. As a long-time EVALIDator user, this was a great way to access FIA data. But dealing with the output in an HTML format and getting it into different software to analyze was always an additional step.
I was happy to learn in the webinar about new ways to directly access FIA estimates through R and Python using the EVALIDator API. For me, this can save time and effort given most of my analyses, graphing, and reporting are done through R.
All of the documentation for these steps can be found in the FIADB-API & EVALIDator user documentation. I’ll go through a few examples where I access data from the state of Maine.
Using R to access FIA data at the state level
This first example will access the total amount of carbon stored in the live aboveground portions of trees growing on forestland in Maine, measured in metric tonnes. I’ll ask for estimates to be provided by forest type group and ownership.
First, I’ll use R and will load a few packages to access the data:
If you look at the bottom of the documentation webpage, you’ll see example use cases for extracting FIA estimates using R and Python. There are two examples for each that use GET and POST scripts. The GET scripts allow you to enter a complete URL if you know the attributes you’re interested in. The POST script, which I copy here with the fiadb_api_POST()
function, allows you to directly input the variables you’re interested in in R:
# fiadb_api_POST() will accept a FIADB-API full report URL and return data frames
# See descriptor: https://apps.fs.usda.gov/fiadb-api/
<- function(argList){
fiadb_api_POST # make request
<- POST(url = "https://apps.fs.usda.gov/fiadb-api/fullreport",
resp body = argList,
encode = "form")
# parse response from JSON to R list
<- content(resp, "parsed", encoding = "ISO-8859-1")
respObj # create empty output list
<- list()
outputList # add estimates data frame to output list
'estimates']] <- as.data.frame(do.call(rbind,respObj$estimates))
outputList[[
# if estimate includes totals and subtotals, add those data frames to output list
if ('subtotals' %in% names(respObj)){
<- list()
subtotals # one subtotal data frame for each grouping variable
for (i in names(respObj$subtotals)){
<- as.data.frame(do.call(rbind,respObj$subtotals[[i]]))
subtotals[[i]]
}'subtotals']] <- subtotals
outputList[[
# totals data frame
'totals']] <- as.data.frame(do.call(rbind,respObj$totals))
outputList[[
}
# add estimate metadata
'metadata']] <- respObj$metadata
outputList[[
return(outputList)
}
The first item to know before accessing FIA data is the numeric code corresponding to the variable you’re interested in. In my case, this is snum = 98
, corresponding to the variable that represents “Forest carbon pool 1: live aboveground, in metric tonnes, on forest land.” A note of caution: there are thousands of variables to choose from, but I suspect the most popular ones are listed toward the top of the page.
The second item to know is which wc code you’re interested in. I have no idea why it’s abbreviated “wc”, but it connects the state FIPS code with the 4-digit FIA inventory year. For example, I’m interested in wc = 232021
to obtain data from Maine’s (FIPS code 23) most recent inventory, collected in 2021. You could go back to inventories collected decades ago if you were interested in looking at trends, and those codes are described here.
Finally, you can use the rselected
and cselected
statements to identify the variables you’d like to group by in rows and columns. In our case this is forest type group and ownership group. I’ll obtain the data in an NJSON format, but you can also obtain the data in HTML, XML, or other formats. You can specify these parameters in arg_list
and then store the data in a data frame called all_rows
. I love how the data are presented in a long and “tidy” format:
<- list(snum = 98,
arg_list_maine wc = 232021,
rselected = 'Forest type group',
cselected = 'Ownership group - Major',
outputFormat = 'NJSON')
# submit list to POST request function
<- fiadb_api_POST(arg_list_maine)
post_data_maine
# estimate data frame
<- post_data_maine[['estimates']]
all_rows_maine
print(all_rows_maine)
ESTIMATE GRP1 GRP2 PLOT_COUNT
1 3904898 `0001 White \\/ red \\/ jack pine group `0001 Public 22
2 34789659 `0001 White \\/ red \\/ jack pine group `0002 Private 248
3 14310434 `0002 Spruce \\/ fir group `0001 Public 110
4 105397247 `0002 Spruce \\/ fir group `0002 Private 1022
5 62478.28 `0005 Loblolly \\/ shortleaf pine group `0002 Private 1
6 200517.1 `0018 Exotic softwoods group `0001 Public 1
7 531124 `0018 Exotic softwoods group `0002 Private 5
8 823381.8 `0020 Oak \\/ pine group `0001 Public 5
9 10607119 `0020 Oak \\/ pine group `0002 Private 73
10 992844.6 `0021 Oak \\/ hickory group `0001 Public 8
11 11742364 `0021 Oak \\/ hickory group `0002 Private 85
12 409060.3 `0023 Elm \\/ ash \\/ cottonwood group `0001 Public 7
13 6009873 `0023 Elm \\/ ash \\/ cottonwood group `0002 Private 85
14 14742425 `0024 Maple \\/ beech \\/ birch group `0001 Public 111
15 136482887 `0024 Maple \\/ beech \\/ birch group `0002 Private 1244
16 3260952 `0025 Aspen \\/ birch group `0001 Public 28
17 29091440 `0025 Aspen \\/ birch group `0002 Private 330
18 5415.465 `0030 Other hardwoods group `0001 Public 2
19 734850.1 `0030 Other hardwoods group `0002 Private 26
20 2668.444 `0034 Nonstocked `0001 Public 1
21 56632.09 `0034 Nonstocked `0002 Private 10
SE SE_PERCENT VARIANCE
1 989847.4 25.34887 979797935531
2 2438499 7.009263 5.946276e+12
3 1346913 9.412105 1.814175e+12
4 3093327 2.934922 9.568672e+12
5 69535.69 111.2958 4835212844
6 197339.8 98.41544 3.8943e+10
7 322121.4 60.649 103762218840
8 383501.6 46.5764 147073471553
9 1405489 13.25043 1.975398e+12
10 376671.9 37.93866 141881751049
11 1392492 11.8587 1.939034e+12
12 210167.8 51.3782 44170517675
13 792626.1 13.18873 628256055243
14 1316485 8.929905 1.733132e+12
15 3598018 2.636241 1.294574e+13
16 719201 22.05494 517250138459
17 1931424 6.639148 3.730397e+12
18 4321.885 79.80635 18678690
19 191250.2 26.02575 36576657832
20 2380.922 89.2251 5668789
21 31032.09 54.79594 962990504
This makes the output easy to plot immediately. Here’s a graph of the output, where you can see the vast amount of carbon stored on private land in Maine, mostly in the spruce/fir and maple/beech/birch forest type groups:
ggplot(all_rows_maine, aes(x = as.character(GRP2),
y = as.numeric(ESTIMATE))) +
geom_bar(stat = "identity") +
facet_wrap(~as.character(GRP1)) +
labs(x = "Ownership",
y = "Forest carbon (metric tonnes)")
Note you’ll need to play with the names of variables a bit to tidy them up, e.g., turning “`0001 Public” to simply “Public”. But the R functions allow you to quickly obtain the data of interest. You can also grab the subtotals of the output to sum all values within each forest type or ownership category:
<- post_data_maine[['subtotals']]
subtotals_maine
print(subtotals_maine)
$GRP1
ESTIMATE GRP1 PLOT_COUNT SE
1 38694556 `0001 White \\/ red \\/ jack pine group 269 2618535
2 119707681 `0002 Spruce \\/ fir group 1127 3344993
3 62478.28 `0005 Loblolly \\/ shortleaf pine group 1 69535.69
4 731641.2 `0018 Exotic softwoods group 6 377174.6
5 11430501 `0020 Oak \\/ pine group 78 1452201
6 12735208 `0021 Oak \\/ hickory group 92 1432097
7 6418933 `0023 Elm \\/ ash \\/ cottonwood group 92 815680
8 151225312 `0024 Maple \\/ beech \\/ birch group 1348 3791540
9 32352393 `0025 Aspen \\/ birch group 357 2057692
10 740265.6 `0030 Other hardwoods group 28 191299.1
11 59300.54 `0034 Nonstocked 11 31123.29
SE_PERCENT VARIANCE
1 6.767193 6.856727e+12
2 2.794301 1.118898e+13
3 111.2958 4835212844
4 51.55185 142260655272
5 12.70461 2.108887e+12
6 11.24518 2.050902e+12
7 12.70741 665333890414
8 2.507213 1.437578e+13
9 6.360247 4.234097e+12
10 25.84195 36595336521
11 52.484 968659293
$GRP2
ESTIMATE GRP2 PLOT_COUNT SE SE_PERCENT VARIANCE
1 38652597 `0001 Public 274 1659512 4.293404 2.75398e+12
2 335505672 `0002 Private 2891 3373908 1.005619 1.138325e+13
Finally, you can grab the total number that sums all values. In this case, we learn that there’s about 374 million metric tonnes of carbon stored in the live aboveground portions of trees in Maine forests:
<- post_data_maine[['totals']]
totals_maine print(totals_maine)
ESTIMATE PLOT_COUNT SE SE_PERCENT VARIANCE
1 374158269 3143 3430416 0.9168357 1.176776e+13
Using R to access FIA data around a fixed geographic point
A favorite use of EVALIDator by many is the ability to query FIA data around a specific location. For example, a user can generate population estimates for a 50-mile radius around a proposed mill that uses wood.
Here’s an example that queries FIA data in a 25-mile radius surrounding Bangor, Maine. It estimates the total forestland area (snum = 2
) by stand age class in 20-year increments and stand size class (large-, medium-, or small-diameter trees). The latitude and longitude coordinates are specified in the function:
<- list(lat = 44.809,
arg_list_bangor lon = -68.771,
radius = 25,
wc = 232021,
snum = 2,
rselected = 'Stand-size class',
cselected = 'Stand age 20 yr classes (0 to 100 plus)',
outputFormat = 'NJSON')
# submit list to POST request function
<- fiadb_api_POST(arg_list_bangor)
post_data_bangor
# estimate data frame
<- post_data_bangor[['estimates']]
all_rows_bangor
print(all_rows_bangor)
ESTIMATE GRP1 GRP2 PLOT_COUNT SE
1 14155.6 `0001 Large diameter `0002 21-40 years 4 7338.611
2 45473.05 `0001 Large diameter `0003 41-60 years 10 15372
3 90436.76 `0001 Large diameter `0004 61-80 years 18 22385.23
4 107109 `0001 Large diameter `0005 81-100 years 23 24368.78
5 41243.83 `0001 Large diameter `0006 100+ years 7 15696.3
6 51611.41 `0002 Medium diameter `0002 21-40 years 12 16379.57
7 113830.6 `0002 Medium diameter `0003 41-60 years 25 24352.08
8 199754.6 `0002 Medium diameter `0004 61-80 years 37 33455.07
9 103521.8 `0002 Medium diameter `0005 81-100 years 21 24195.48
10 5758.7 `0002 Medium diameter `0006 100+ years 1 6011.665
11 19655.8 `0003 Small diameter `0001 0-20 years 4 10104.88
12 90910.76 `0003 Small diameter `0002 21-40 years 16 23393.71
13 40205 `0003 Small diameter `0003 41-60 years 7 15304.59
14 13209.02 `0003 Small diameter `0004 61-80 years 3 8759.869
15 21404.85 `0003 Small diameter `0005 81-100 years 3 11056.3
16 5305.999 `0003 Small diameter `0006 100+ years 1 5045.363
SE_PERCENT VARIANCE
1 51.84246 53855209
2 33.80465 236298512
3 24.75236 501098555
4 22.75138 593837455
5 38.05733 246373784
6 31.73633 268290228
7 21.39326 593023809
8 16.74808 1119241412
9 23.37235 585421221
10 104.3927 36140117
11 51.40919 102108689
12 25.73261 547265614
13 38.06637 234230329
14 66.31735 76735306
15 51.65323 122241669
16 95.0879 25455691
You can plot these data directly to visualize the trends within the 25-mile radius:
ggplot(all_rows_bangor, aes(x = as.character(GRP2),
y = as.numeric(ESTIMATE),
fill = as.character(GRP1))) +
geom_bar(stat = "identity") +
labs(x = "Stand age",
y = "Forestland area (acres)") +
theme(axis.text.x = element_text(angle = 45, hjust = 1))
Conclusion
The new features available in the EVALIDator API that allow you to access data through R or Python can help to speed up data analysis using FIA data. You can access the entire history of FIA data and analyze forest resources data by state or a circular retrieval from a fixed geographic point. Special thanks to the USDA Forest Service staff that have made this available!
–
By Matt Russell. Email Matt with any questions or comments.